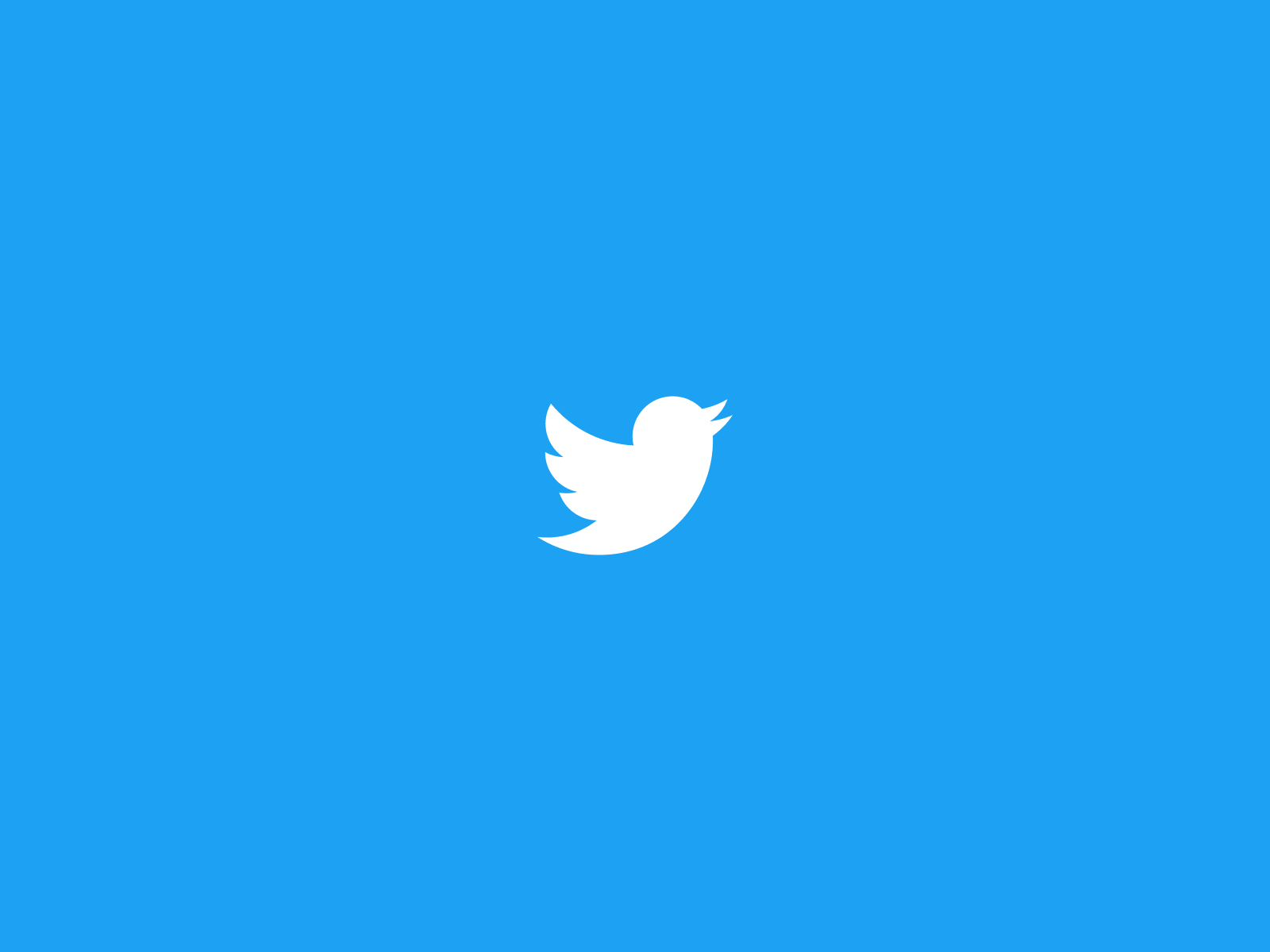
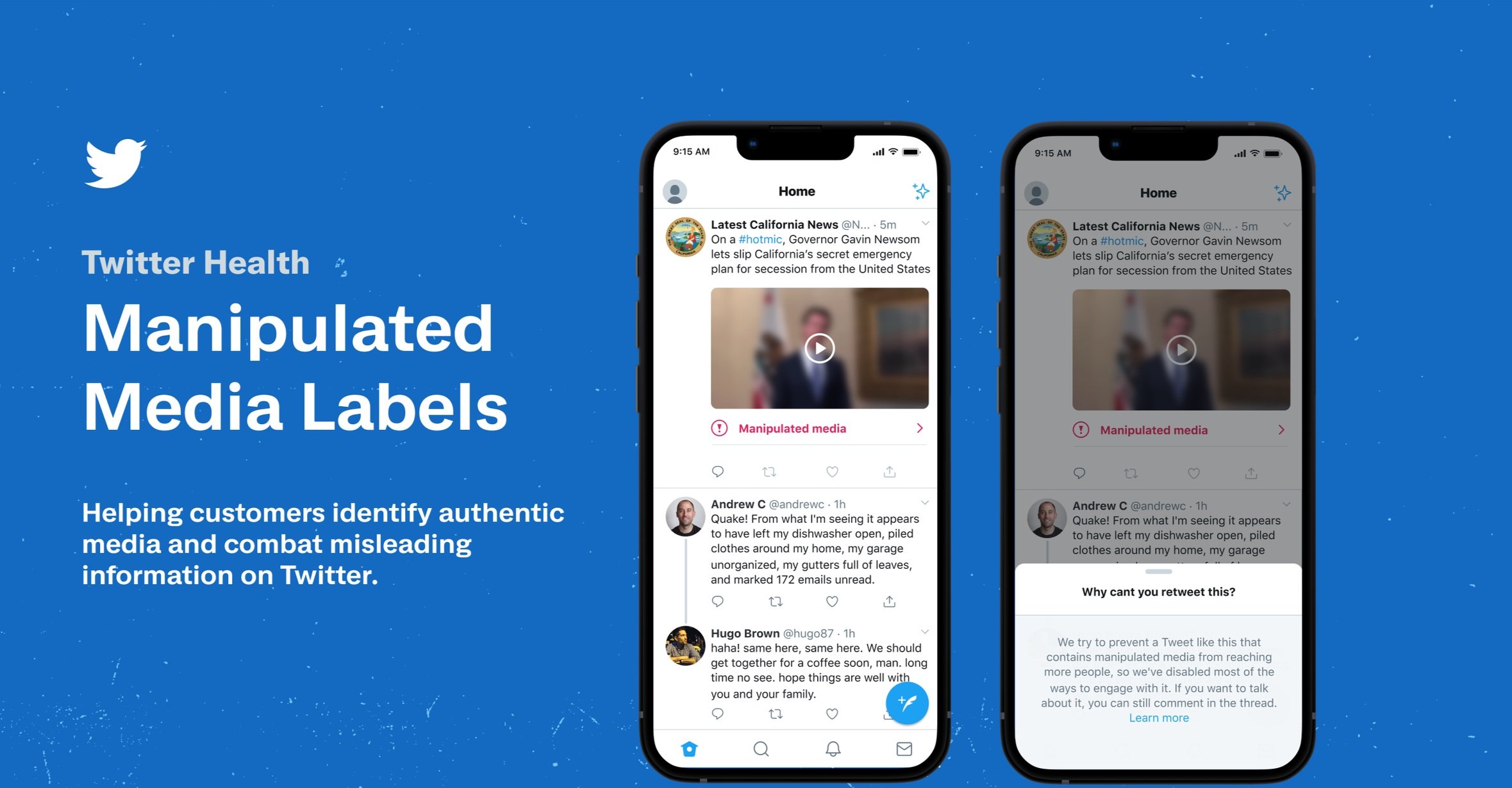
Case Study: Twitter’s Health Manipulated Media Labels
Introduction
In an era of widespread misinformation, particularly concerning health-related topics like COVID-19 and election integrity, Twitter recognized the urgent need to address the proliferation of manipulated media. This case study outlines the design process and implementation of health manipulated media labels aimed at informing users and promoting accurate information.
Problem Statement
The rapid spread of manipulated media poses significant risks to public health and democratic processes. Users often encounter misleading content without adequate context, which can lead to confusion and mistrust. Our objective was to design and implement effective labels that clearly identify manipulated media, guiding users to reliable information sources.
Research
User Behavior Analysis
We analyzed user interactions with tweets that contained manipulated media, observing patterns in engagement and response. Key insights included:
Users often struggled to discern the reliability of the media.
There was a notable demand for more transparency and context regarding shared content.
Expert Consultation
We engaged with health and misinformation experts to understand the nuances of manipulated media. Their insights helped shape our approach to labeling and providing context.
Competitive Analysis
An examination of other platforms’ strategies revealed various approaches to misinformation labeling. Successful implementations often featured clear visual cues and informative links to authoritative sources.
Design Process
1. Define User Personas
We created user personas representing various demographics, including:
Concerned citizens seeking accurate health information.
Political users engaged in discussions around elections.
Casual users who might inadvertently share manipulated content.
2. Ideation and Concept Development
Brainstorming sessions generated several concepts for media labels:
Visual labels with distinct colors and icons to signify manipulated media.
Tooltip explanations that provide context when users hover over the label.
Links to authoritative sources for users to verify information.
3. Wireframing
Wireframes were developed for how the labels would appear on tweets. Key features included:
A clear label design (e.g., “Manipulated Media” with an icon).
Contextual information available on hover or click.
Examples of similar media for users to understand the implications.
4. High-Fidelity Prototyping
Using Figma, we created high-fidelity prototypes of the tweet interface, incorporating the new labels and interactive elements. This allowed for visual testing of the label’s impact on user experience.
Implementation
Collaboration with Development
We worked closely with the development team to integrate the labels into the existing Twitter architecture. This involved ensuring compatibility across platforms and devices.
User Testing
We conducted user testing sessions to evaluate the effectiveness of the labels. Participants were asked to interact with tweets containing manipulated media and provide feedback on their understanding and trust levels.
Results
The implementation of health manipulated media labels yielded promising outcomes:
Increased Awareness: Users reported a heightened awareness of manipulated content, with a 35% increase in recognition of labels compared to previous experiences.
Trust Levels: Surveys indicated a 25% increase in users’ trust in the information presented alongside labeled content.
Engagement with Resources: There was a significant uptick in clicks to authoritative sources linked in the labels, suggesting that users were seeking to verify information.
Conclusion
The design and implementation of health manipulated media labels on Twitter effectively addressed the challenges of misinformation, enhancing user awareness and encouraging responsible sharing practices. By providing clear visual cues and access to reliable sources, Twitter took significant steps toward fostering a more informed user base.
Future Considerations
To build on the success of this initiative, future iterations could explore:
Expanding label functionality to include user-generated content warnings.
Incorporating machine learning to improve the identification of manipulated media.
Developing educational campaigns to inform users about recognizing misinformation.
By continually refining these tools, Twitter can further strengthen its role in combating misinformation and promoting public trust.